While the development of ChatGPT continues to evolve rapidly, there is an equal rise in headlines declaring that AI perpetuates racial and sexist stereotypes. Kaiping Chen and her team investigated how GPT-3 fared in conversations on controversial science topics as well as other social issues. In our interview, she shares their discoveries.
Is generative AI biased?
You looked at user experience with GPT-3 discussing science topics. We frequently hear that “AI is biased”. Is this something that you observed in your preprint?
In my work, I tend to use the word “equity” instead of bias because “bias“ tends to narrow down our examination of AI to certain aspects such as gender, race and ethnicity. There are other key aspects how generative AI converses with people with different values and cultural backgrounds, which are issues that the word bias cannot summarize well. An AI can be biased in certain dimensions, but they might play a positive role in other aspects. Equity allows us to examine these competing forces of AI.
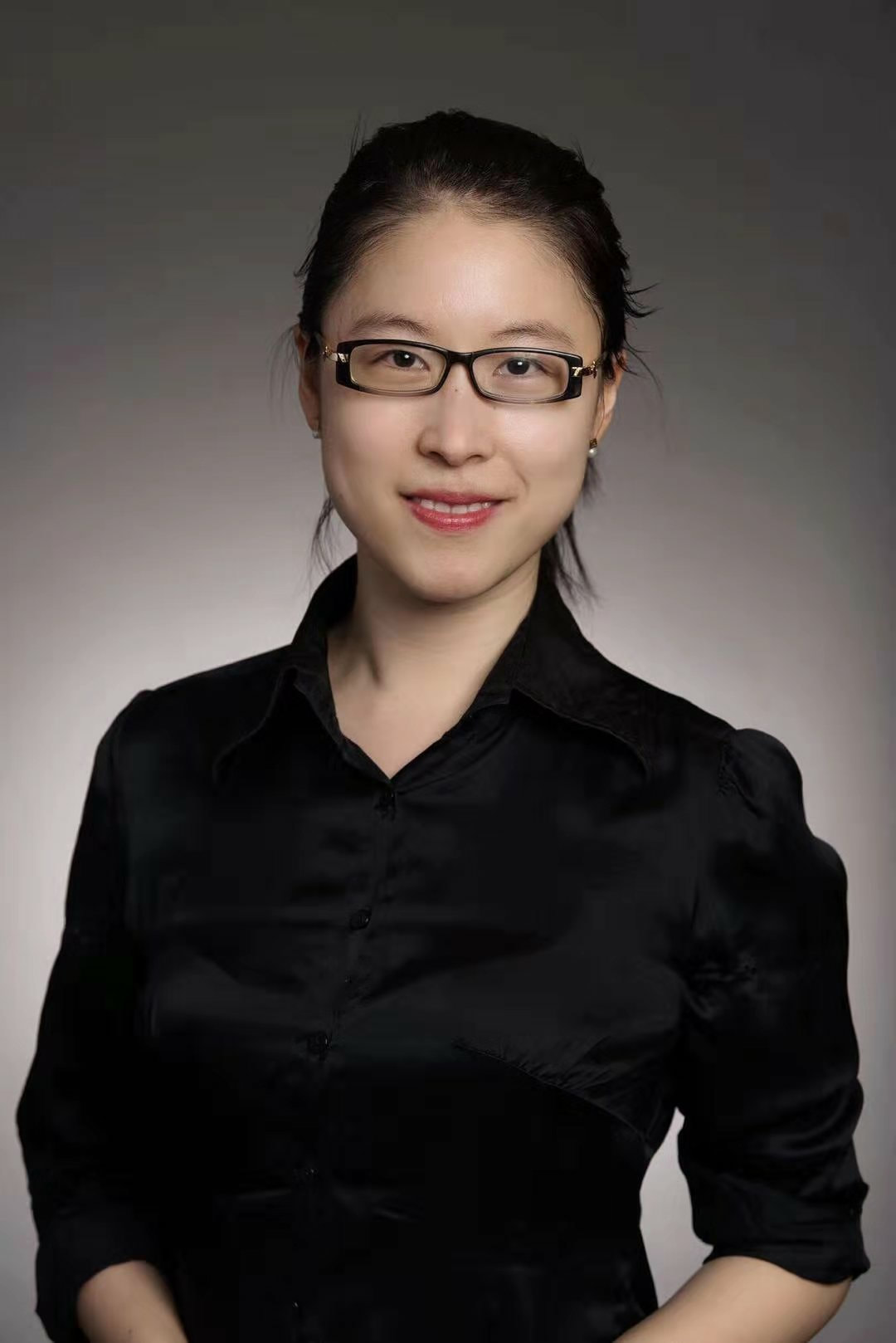
In this study, for example, I had individuals from diverse social backgrounds interact with GPT — bearing in mind this study predates the current GPT version by two years — the quality of conversation emerged as a key aspect. We found that female and male participants, participants of different race and ethnicity – their user experience after chatting with GPT were quite similar. But there was a significant difference among the people who we call “opinion and education minorities”.
Could you elaborate?
These opinion minorities, which were 10 to 15 percent of the 3,000 participants, held different perspectives on subjects like climate change and Black Lives Matter. They don’t believe in or doubt climate change or are not supportive of the Black Lives Matter movement. The education minority group had a highschool degree or lower. Both opinion and education minority groups had a stronger aversion to chats with GPT-3 compared to their counterparts.
We probed participants‘ viewpoints both before and after conversing with GPT-3. This allowed us to measure attitude shifts. The interesting thing we found was that despite expressing more dissatisfaction about the conversation, minority groups showed a positive shift in their attitudes, particularly regarding climate change. So they don’t really support the climate initiative, but compared to before, they are more supportive. This is why I don’t call it bias. You might say that the user experience is not equal between opinion majority and opinion minority groups. The latter have a less enjoyable user experience, but they are learning. How user satisfaction and learning interact when it comes to these controversial topics is something we are keen to keep exploring in the future.
You also found GPT used different rhetorical strategies depending on the subject. How did this affect the opinion and education minority groups?
We looked at the use of evidence by the AI comparing the two subjects. Use of evidence can be conceptualized very broadly. Citing external links, like Wikipedia, citing scientists or the use of storytelling. What we found is that when GPT-3 talked to an opinion minority about climate change, it actually tried to share a lot of evidence. But when it came to the Black Lives Matter movement, we saw that when talking to the opinion minority the AI used a preference-based response. It basically said: “I don’t agree with you”. It doesn’t really cite evidence. So what we observe is that there is a difference across the two issues. We can’t say that an AI-based conversation is biased when people talk to them about every topic. The AI might excel at movie recommendations but falter on specific subjects.
You write that “inequality is always in the room” because different languages carry different cultural powers. How can AI development take this complexity into account?
This is something my team and I are working on right now. We look into how GPT talks to people who speak Spanish, who come from different Latinx cultures. Most of the data AI is based on is trained on the English internet. But when it comes to relatively underrepresented languages, how will GPT respond? Will it give weird answers? We are hiring people from different Latinx cultures outside and within the US asking them to use their preferred language to talk to ChatGPT. Then we compare the quality of the responses to people whose native language is English.
One of my team members shared an anecdote, where ChatGPT described a taco recipe in a distinctly Americanized way rather than the Latino version. This highlights the importance of recognizing cultural nuances. So when we think about a conversational AI system, we need to be attentive to the local context, cultural nuances and the issues that resonate with a particular audience. It’s not solely about the type of knowledge you are sharing with people, but also fundamentally, do you really recognize their culture? Equitable AI systems should capture and honor cultural intricacies.
Should there be – as opposed to industrial proprietorship – publicly funded development of AI tools?
There are two key points to address here. From the researcher’s standpoint, algorithm auditing plays an important role. Researchers want to demystify the black box, looking for a deeper understanding through user interfaces and data access. The more intricate facet involves the wider ecosystem, encompassing companies, researchers, and regulators. This taps into a larger concern – the collaborative framework required for transparency. While initiatives like Open AI’s grant calls for democratic input are steps in the right direction, the core question remains: What shape should this transparent system take? And who ensures that transparency and open-sourcing are upheld rather than being driven by specific researchers or companies?
This requires a comprehensive system. Firstly, funding mechanisms for such research need careful consideration. Secondly, and more importantly, who is the entity responsible for ensuring transparency in AI development? Ideally, the public needs to be involved, not just the one public or a certain public, but the publics, the plural form. It’s those people who share a different perspective, who have different opinions about the issue. They need to be involved, engaged into this whole conversation of how we build this system.
You propose a framework to audit equity in conversational AI that may also be used to audit the later versions of GPT. Could you explain what we need to keep in mind when auditing AI systems regarding equity?
Technology is evolving rapidly. Within the two years since we conducted the research, we saw Chat-GPT, GPT-3, GPT-4. And we researchers have some catching up to do. Our findings show that we need to go beyond the conventional discussions of gender and race and explore how systems communicate with individuals holding diverse values, attitudes, and perspectives. As technology advances, so too must our strategies to ensure a harmonious intersection between user engagement, education, and evolving AI dynamics.
Our auditing framework centers around three important pillars: diversity in who is engaged in the system creation and assessment, comparability in user experience and learning across different groups as well as comparability in the use of evidence styles towards different groups. Our framework includes the process from inviting participants to the table to scrutinizing dialogue nuances and finally evaluating user experiences and learning. It’s a dynamic process, emphasizing the interplay between diversity, education, and conversation – something we should keep in mind if we are investigating equitable and effective human-computer interaction.
Further reading
Chen, K., Shao, A., Burapacheep, J., & Li, Y. (2022). A critical appraisal of equity in conversational AI: Evidence from auditing GPT-3’s dialogues with different publics on climate change and Black Lives Matter. arXiv preprint: 2209.13627.